Tech
Jacopo Chiapparino
Jan 13, 2022
Why a Data Platform? The role of Data & Insights at Wolt
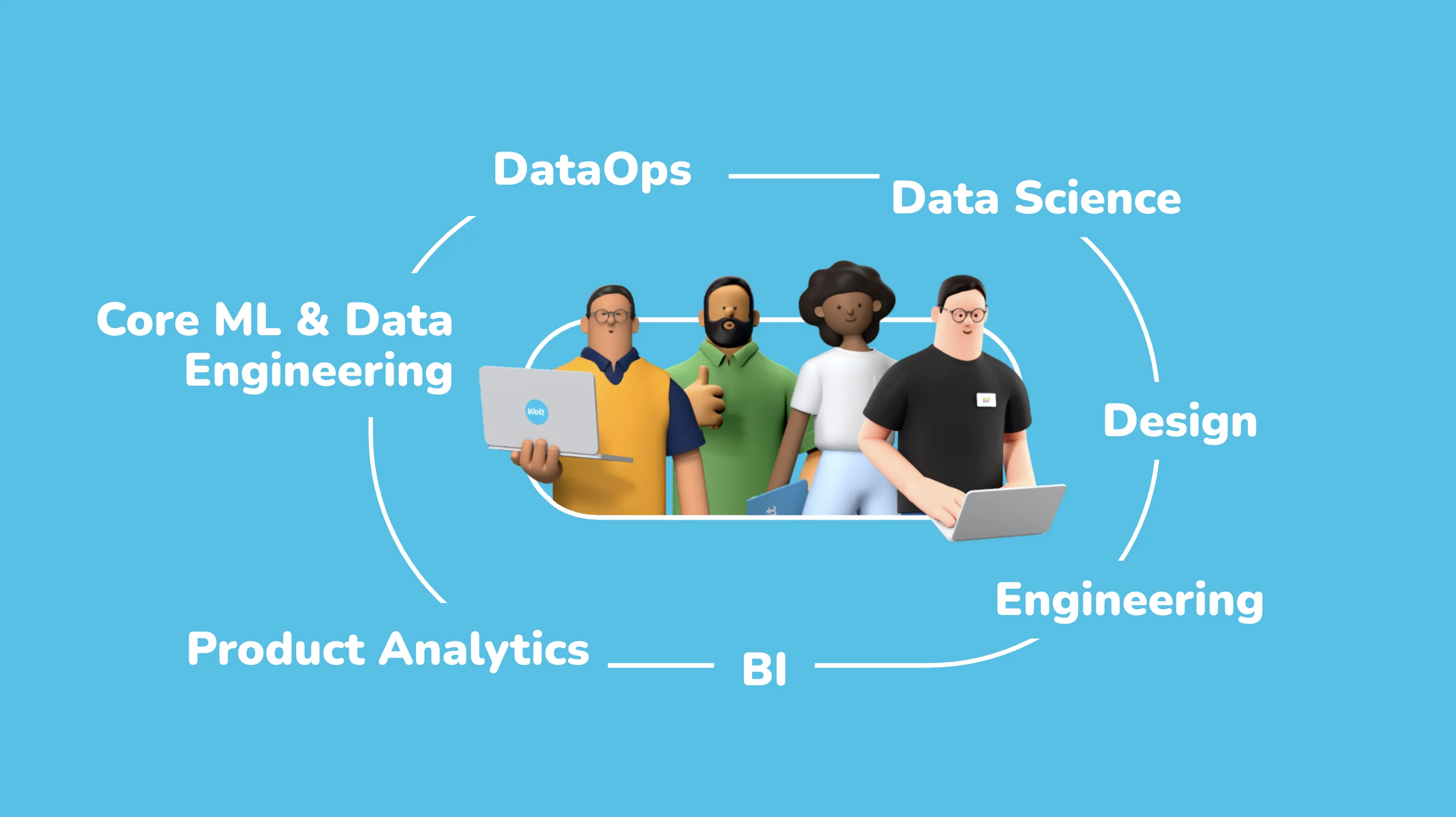
Data Platforms are an essential part of modern businesses. They enable reporting, low friction decision making, and if used correctly, can power very advanced data products in a compliant and traceable manner.
Let us walk you through our core data platform at Wolt and its use cases in this series of blog posts. In this first one we’ll take you from the role of data at Wolt, through the data journey we’ve had so far and finish with a peek into what the future of this discipline may look like, enjoy!
The role of data and insights at Wolt
Gathering data is a complex problem. At Wolt data is never treated in isolation — it’s part of a bigger and deeper multidisciplinary practice which aims to empower people to run our business more effectively. We gather data primarily to recreate our global context in one place. This allows the entire company to tap into a single source of truth for the more disparate use cases: from running an analysis in one of our operations teams to building new product features.
At the core of our data is the information produced by Wolt users interacting with a product feature, like the search feature on Wolt.com, built by one of our product teams. As this data gets harvested and collected in our warehouse, we contextualise it and build relationships with other datasets, originating from different product features maintained by other product teams. Ultimately this results in the global context which constitutes the foundation of any insights.
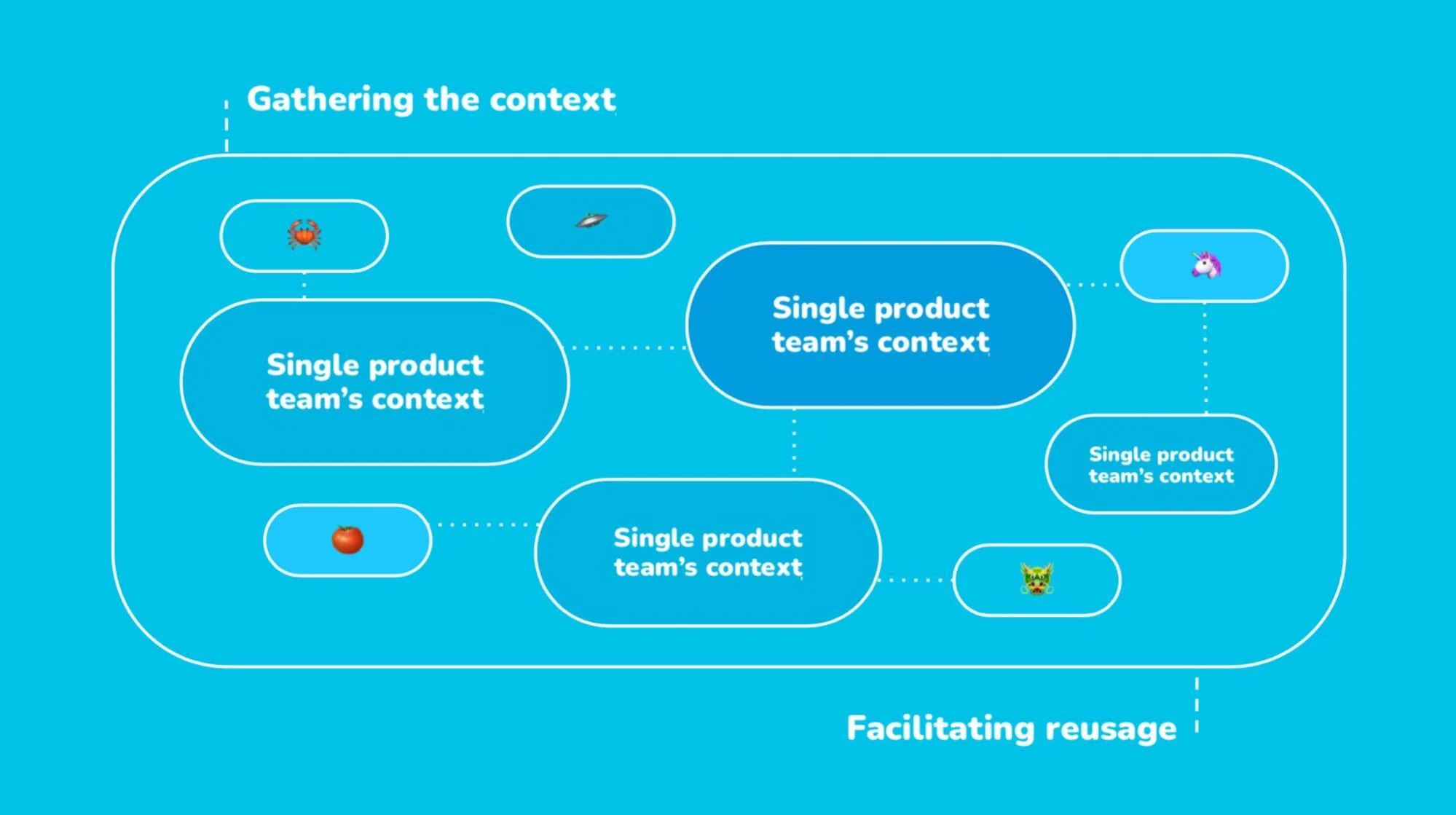
Since the early days we’ve grown fond of data and have practised running our business by putting everyone in close contact with our data tools. Everyone at Wolt can to tap into the global context of our business. Throughout the years our teams have learned to talk about our business using the same terminology, regardless of the business unit, country or role.
At Wolt, we’ve grown from a handful of product teams into 35+ teams in the span of just a few years. Meanwhile, the core of our data engineering team has remained quite small and efficient. We put a lot of effort into educating every product team on the importance of the data they’re gathering and turning data around from being a byproduct into something that can power insight-driven products across our organisation.
We’re continuously developing the means for our data to become organised and well-presented information that allows teams to draw insights in easy, reproducible and compliant ways. The ultimate goal is to build increasingly smart data products.

Journey to data maturity
As said above, we have many teams collaborating together to keep making Wolt a more data and insight driven company. We’re far from having a perfect, well-oiled machine, so there’s still loads to do. I’d like to think we’re still on a journey towards “data maturity”, and still in the process of becoming fluent with the different aspects of working with data.
Once we reach this maturity, it will mean that the practises around data collection, analysis and utilisation of data in data science will be as comfortable for our teams as it is for them to build and release our services and applications.
In order to accelerate and guide this journey we’ve undertaken several initiatives starting from 2019 to first of all build the right environment to carrying out successful data projects and advanced analytics practises:
To create the right environment we kickstarted a team in 2019 called Core Machine Learning and Data Engineering (CMLD) to specifically build the core of our data engineering platform. All of the core pieces of our data infrastructure are currently developed under this team’s umbrella: data gathering and collection, quality monitoring, experimentation and the machine learning infrastructure.
We’ve started building our Business Intelligence, Analytics and Data Science disciplines centrally, as close as possible to our data stack. This has helped us ensure that the feedback loop between the professionals building our core data tooling and the ones utilising it is as short as possible. In the past few years we’ve invested a lot in what we call “data bootcamp”, to help lower the barrier between analytics and data engineering.
We realised that these data and analytics disciplines can’t remain isolated in central teams: hoping that product teams would become interested in these skills and offering is just not enough. To drive this change we started to embed our analysts and data scientists into our teams and formed a new team called Data Operations (DataOps) to further lower the barrier between the product teams and the data and analytics disciplines.
During the course of 2022 our Analysts, Data Scientists and DataOps Engineers will start to work more closely together within our product teams to drive this narrative shift from data as byproduct to data as a real opportunity. This will open the door for new, smarter products. All of this will require scaling our data platform to support more use cases, more professionals and more load on its components driven by the increased data collection and processing requirements.
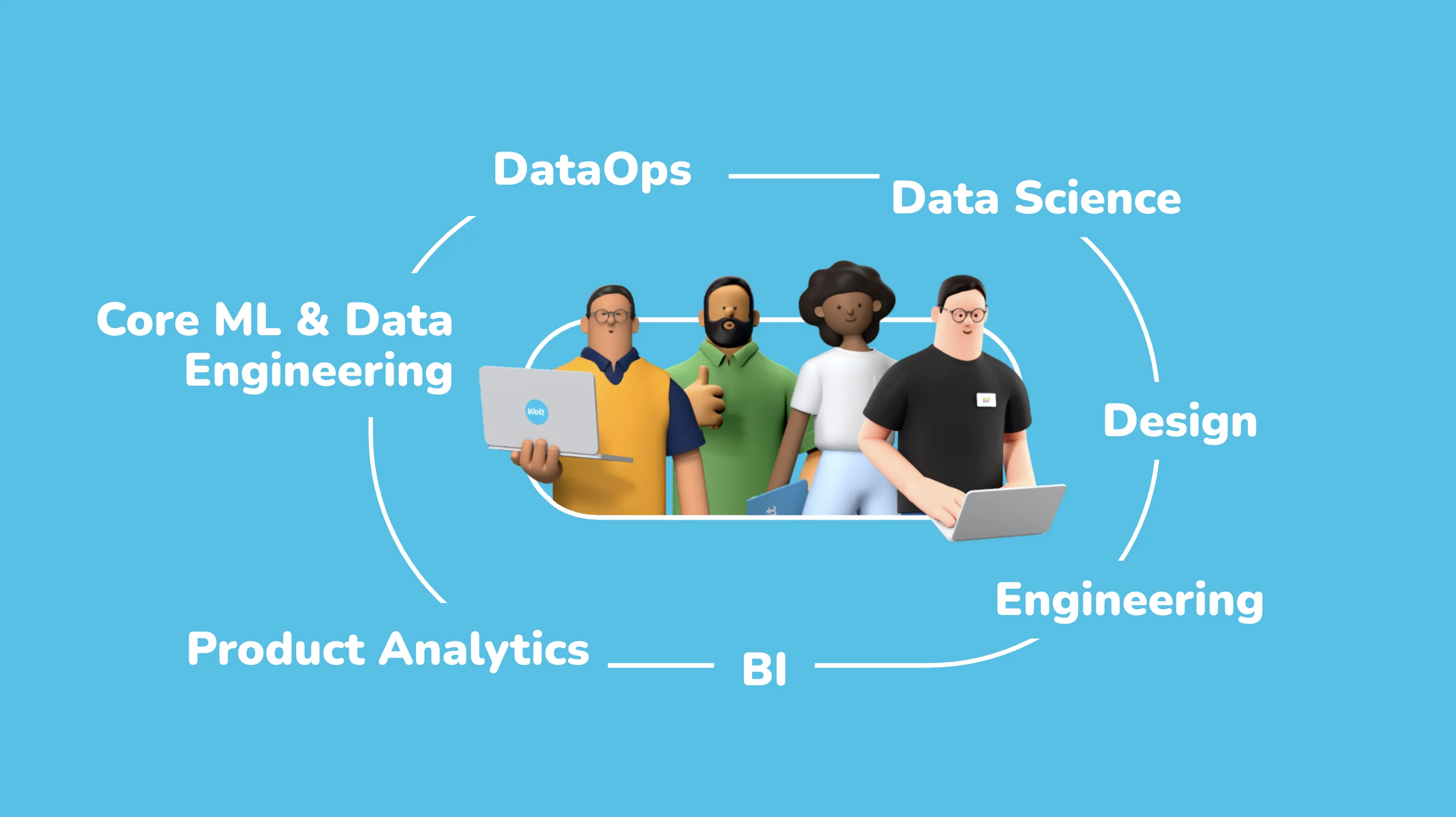
Early days, towards strategic decision making
Before we dive deeper into our data platform itself, let’s look back to see how we got to this point. From the beginning we’ve run our business on top of our data and put a lot of attention to the ergonomics of it. Being able to learn and iterate our business model fast has been important for our decision making from our very first city. Data is crucial for us to understand how our customers and partners interact with our apps, and how to keep improving.
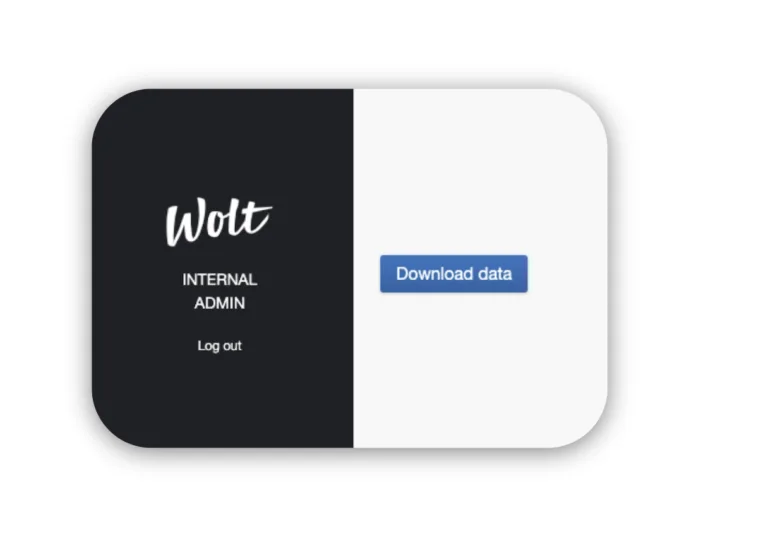
In the early days this meant having a button in our internal tools to integrate our purchase data in a safe way into our first BI tool… our Excel data playground. It might not sound like an extremely advanced solution, however at that stage of our company’s data journey we were focused on ensuring we could iterate fast on our KPIs, in a bid to improve the efficiency of our operations as fast as possible.
This is what I consider our crawling phase: we started to catalogue data and to value it as both an asset and as a discipline. Many of the metrics we track today have been conceptualised at this stage of our data journey, which lasted until early 2016.
Having outgrown this direct integration, during the remaining part of 2016 we started to establish our data warehouse and ETL practises. The core of this early work remains in use today!
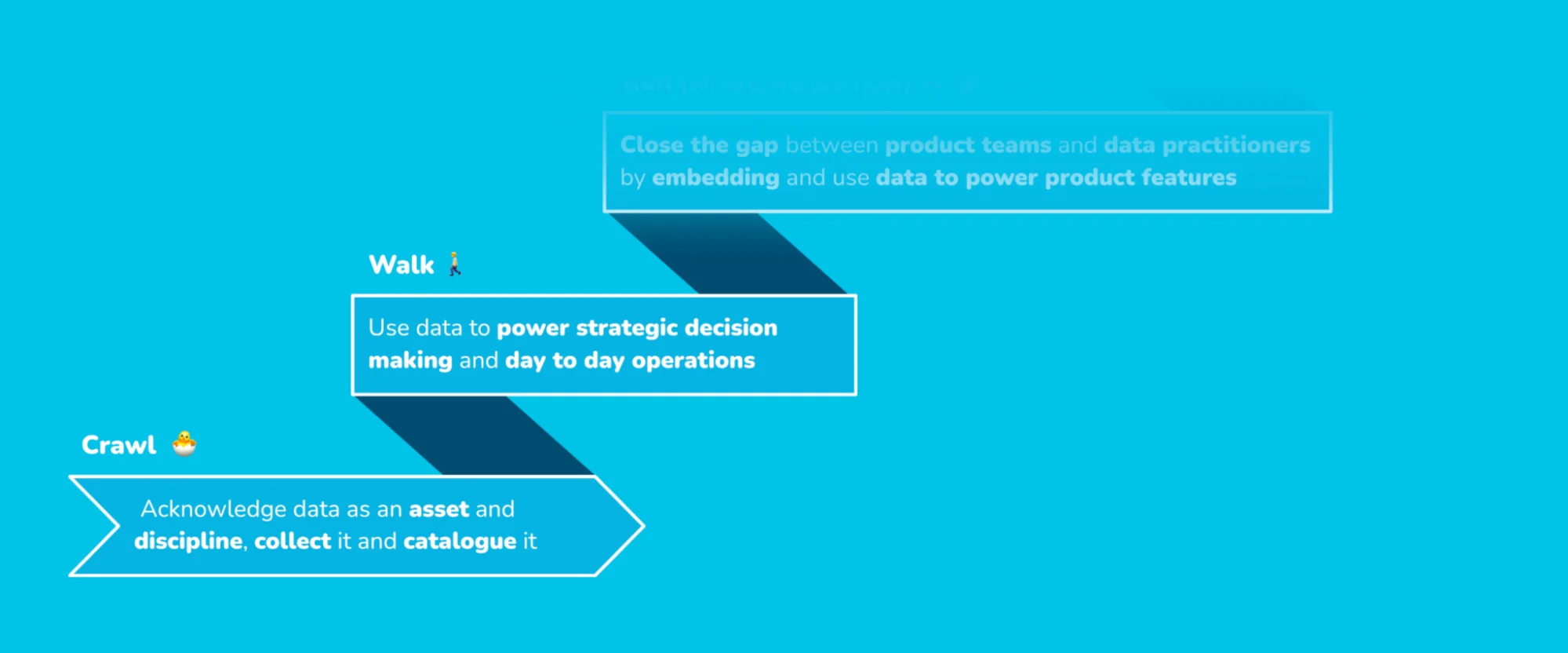
The multi-service based approach that our backend at Wolt was built with, steered our decision to primarily build our data platform around two components: a data integration service and a primarily operational-data-store type of data warehouse. Back in the early days the platform was completed by one or more data exploration tools, used by teams to dive into our relational model, and plot, share and extract data to power different types of analysis.
I consider 2021 pretty much the end of this phase of discovering how our data fits into our operations optimisation and product development. Our data practitioners have learned to work with our platform, which in turn has evolved and matured a lot from the early days.

Looking into the future — chasing insights and powering data products
Now we’re looking into scaling our data platform utilisation from a handful of teams to all of our teams, from a handful of use cases to multitude of use cases, from it powering mostly our operations optimisation, to powering product development and features. Ultimately, from basic analytics to more advanced, complex studies.
Until this point in time we’ve talked about data mostly from the data gathering angle. We’re now moving into more advanced, different means of unlocking deeper data utilisation across our product organisation. As part of our journey to maturity we’ve already seen that one of the big changes in this domain will be driven by continuously embedding more of our analytics and data professionals directly into product teams, to foster a deeper collaboration. This has already proven useful in identifying lots of new and interesting opportunities to collaborate on and will be the direction from now on.
Ultimately we believe that the role of a modern data platform is to foster data-driven product development and to ensure that continuous learning can happen in a safe, metadata rich and compliant environment.
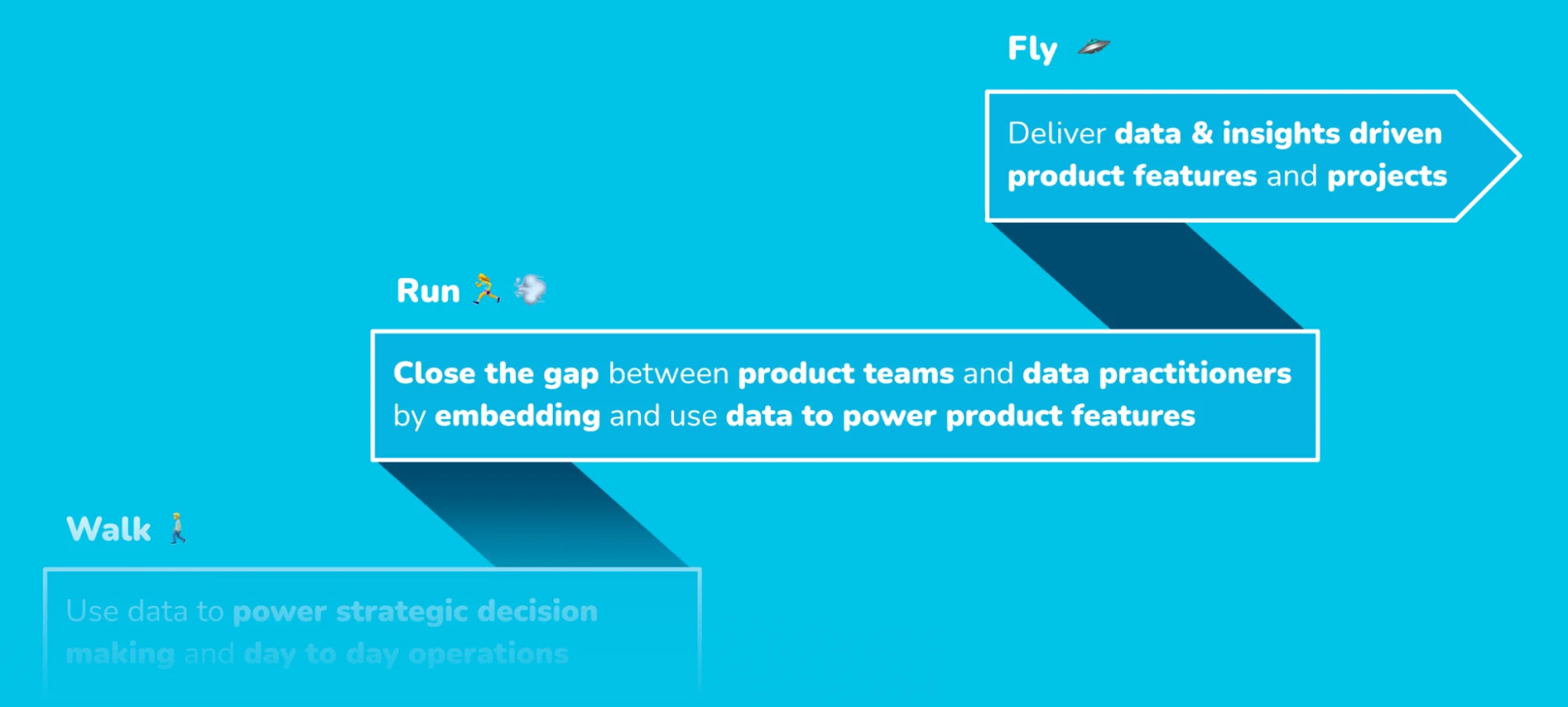
Stay tuned for the next chapter of this series introducing the components of our Data Platform.